Unlocking the power of data science analytics has become a crucial aspect of businesses in today’s digital age. In a world where every decision is driven by data, having the ability to analyze and interpret large volumes of data can give businesses a competitive edge. With the exponential growth of data, it has become more important than ever to harness its power through advanced analytical techniques. This comprehensive guide will provide insights on how businesses can unlock the full potential of data science analytics.
How Data Science Analytics Works
Data science analytics involves the extraction of meaningful insights from vast amounts of data using techniques such as predictive modeling, machine learning, and data mining. It utilizes a combination of statistical analysis, programming, and visualization tools to discover patterns and trends within the data. This process starts with identifying business problems that require data-driven solutions. Next, data is collected from various sources and preprocessed to ensure its quality and accuracy. The data is then analyzed using algorithms and models to gain insights and make predictions. Finally, these insights are communicated through visualizations and reports to inform decision-making.
Steps Involved in Data Science Analytics
- Understanding Business Objectives: The first step in data science analytics is to identify the problem that needs to be solved or the goal that needs to be achieved.
- Data Collection and Preprocessing: Once the objectives are clear, relevant data needs to be collected and cleaned for analysis.
- Exploratory Data Analysis: This involves examining the data to identify patterns, correlations, and outliers that may affect the analysis.
- Model Building and Evaluation: Different models are built and tested to find the one that best fits the data and provides accurate predictions.
- Interpretation and Communication: The insights gained from the analysis need to be effectively communicated to stakeholders for informed decision-making.
Benefits of Data Science Analytics
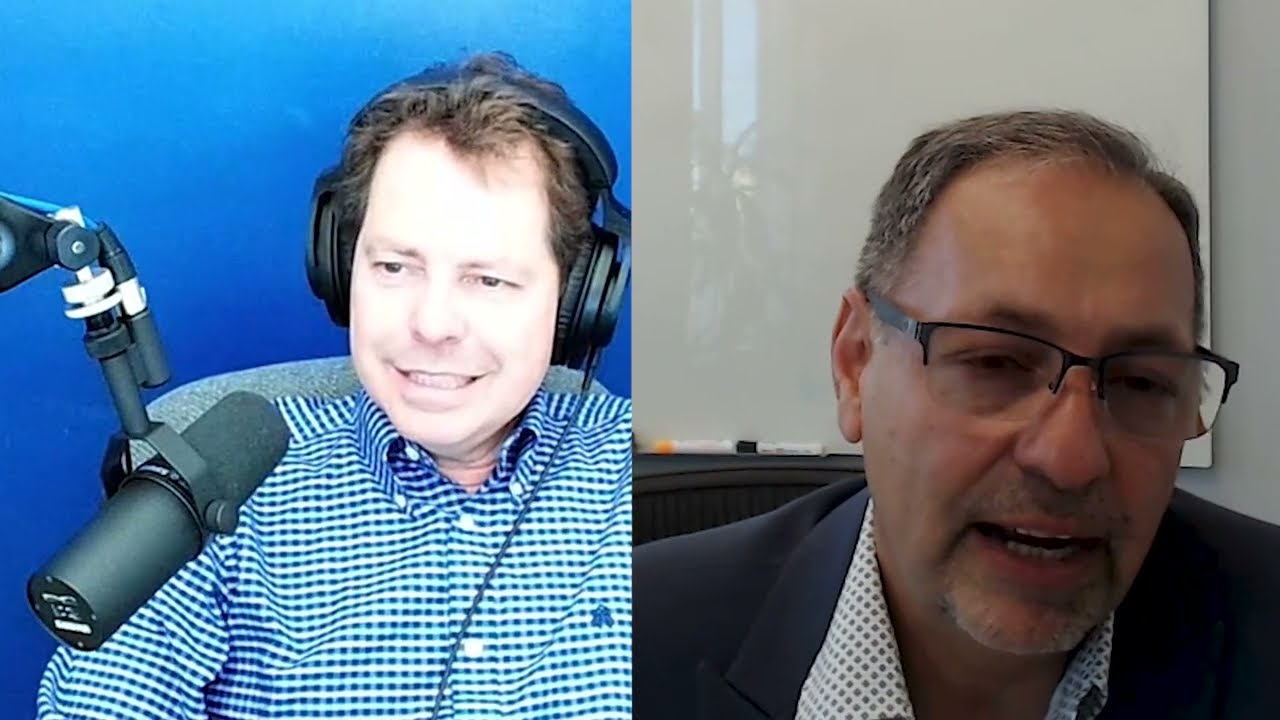
The sheer volume and variety of data available today make it impossible for businesses to ignore its potential. Here are some of the benefits that data science analytics offers:
1. Enhanced Decision-Making
Data science analytics helps businesses make informed decisions based on real-time data. By analyzing past trends and patterns, businesses can determine which strategies have been successful and make data-driven decisions for future initiatives.
2. Improved Customer Insights
With data science analytics, businesses can gather insights into customer behavior and preferences. This information is crucial for understanding customer needs and optimizing marketing efforts to improve customer satisfaction and retention.
3. Cost Savings
By leveraging data science analytics, businesses can identify areas where costs can be reduced and efficiency can be improved. This could be through optimizing supply chain operations, reducing wastage, or increasing productivity.
4. Competitive Advantage
In today’s highly competitive business landscape, data science analytics provides a competitive edge by enabling businesses to stay ahead of market trends and consumer demands. It allows businesses to quickly adapt to changing market conditions and make proactive decisions rather than reactive ones.
How to Use Data Science Analytics
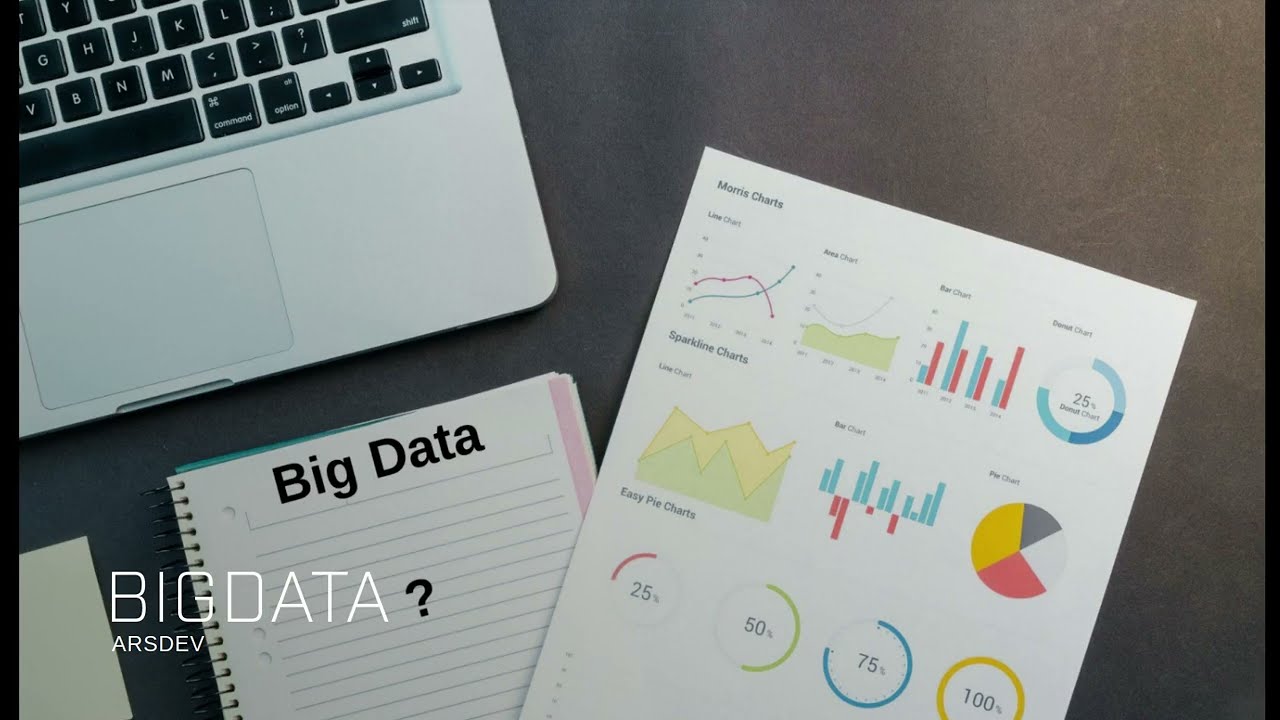
Businesses can utilize data science analytics in various ways, depending on their objectives and industry. Here are some common applications of data science analytics:
1. Predictive Modeling
Predictive modeling is used to forecast future outcomes based on historical data. This technique is useful for identifying customer churn, predicting sales, and detecting fraud.
2. Recommendation Systems
Recommendation systems use data science algorithms to provide personalized recommendations to customers based on their browsing behavior, purchasing history, and preferences. This can lead to increased customer engagement and sales.
3. Sentiment Analysis
Sentiment analysis involves analyzing social media and customer feedback to gain insights into customer opinions and attitudes towards a brand or product. This information can help businesses improve their products and services to better meet customer needs.
4. Fraud Detection
Data science analytics can be used to identify anomalous behavior and detect fraudulent transactions. This helps businesses reduce financial losses and protect their customers’ data.
Real-World Examples of Data Science Analytics
Many companies have successfully leveraged data science analytics to improve their operations and increase revenue. Here are some real-world examples:
1. Netflix
Netflix uses data science analytics to recommend personalized content to its users. By analyzing viewing patterns and preferences, they are able to provide a customized user experience, resulting in increased engagement and retention.
2. Amazon
Amazon utilizes data science analytics to optimize its supply chain operations. With advanced forecasting models, they are able to determine stock levels, reduce wastage, and improve delivery times.
3. Uber
Uber’s surge pricing is a result of data science analytics. By analyzing ride requests and traffic patterns, they are able to set prices based on demand and supply, maximizing their revenue.
4. Walmart
Walmart uses data science to optimize inventory management by analyzing sales data, weather patterns, and market trends. This has resulted in significant cost savings and improved efficiency.
Data Science Analytics vs. Traditional Analytics
While both traditional and data science analytics aim to extract insights from data, there are some key differences between the two:
1. Volume of Data
Traditional analytics involves analyzing structured data, usually from a single source. On the other hand, data science analytics deals with massive volumes of structured and unstructured data from various sources such as social media, sensors, and customer feedback.
2. Predictive Capabilities
Data science analytics has the ability to predict future outcomes and trends, whereas traditional analytics focuses on providing insights into past events.
3. Automation
Data science analytics is highly automated, utilizing machine learning algorithms and artificial intelligence to analyze and make predictions. Traditional analytics, on the other hand, requires manual input and analysis.
4. Scalability
Traditional analytics is limited by the size of the data that can be processed, whereas data science analytics can handle large volumes of data and scale as needed.
Tips for Unlocking the Power of Data Science Analytics
Here are some tips to help businesses maximize the potential of data science analytics:
1. Define Clear Objectives
Before embarking on any data science project, it is crucial to define clear objectives and identify the problem that needs to be solved. This will ensure that the analysis is focused and delivers actionable insights.
2. Invest in Quality Data
Data is the foundation of any data science project, and it is crucial to ensure its quality and accuracy. Investing in data cleaning and preprocessing will result in more reliable insights.
3. Continuously Learn and Adapt
Data science is an ever-evolving field, and businesses must stay updated with the latest advancements and techniques to unlock its full potential. Continuous learning and adaptation are essential for success in this field.
Frequently Asked Questions (FAQs)
Q: What is data science analytics?
A: Data science analytics involves extracting insights from large volumes of data using advanced analytical techniques such as predictive modeling and machine learning.
Q: How does data science analytics work?
A: Data science analytics involves identifying business problems, collecting and preprocessing data, analyzing the data using algorithms and models, and communicating the insights gained.
Q: What are some benefits of data science analytics?
A: Data science analytics offers enhanced decision-making, improved customer insights, cost savings, and a competitive advantage.
Q: How can businesses use data science analytics?
A: Some common applications of data science analytics include predictive modeling, recommendation systems, sentiment analysis, and fraud detection.
Q: What are some real-world examples of data science analytics?
A: Companies such as Netflix, Amazon, Uber, and Walmart have successfully leveraged data science analytics to improve their operations and increase revenue.
Conclusion
Data science analytics has become an essential tool for businesses looking to stay competitive in today’s data-driven world. By understanding the steps involved, the benefits, and how to use it effectively, businesses can unlock the full power of data science analytics. With the right approach and continuous learning, data science analytics can help businesses gain valuable insights, make informed decisions, and drive success.